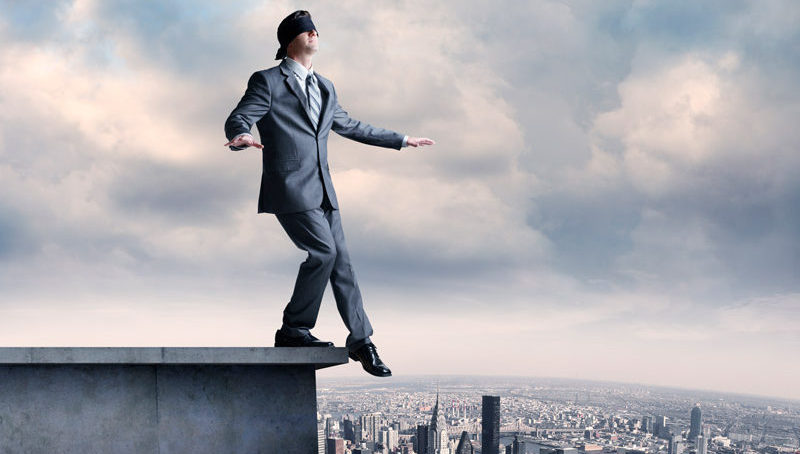
A recent McKinsey report highlighted that only 5% of businesses worldwide have successfully incorporated artificial intelligence (AI) into their processes and offerings.
Despite enthusiastic adoption and significant financial investments, many AI implementations are failing.
One would think that with the ubiquity of AI as a topic and the emergence of companies claiming to specialize in AI, this wouldn’t be the case.
Every single day, more and more data is created, and it is critical to be able to disseminate that data quickly to make AI implementations more accurate and effective. With all of the hype around AI, why does so much of it fail? Easy. Because it isn’t being used to solve a real business problem.
Whether it’s for financial services, healthcare, HR, marketing, tech, or any other vertical, the solution to many business problems can be found in AI, but instead, most AI initiatives do little more than rack up exorbitant costs. Here are three of the main reasons why:
- Lack of Business Alignment
Many executives and IT departments get excited about what AI can bring to their company. However, most organizations that are throwing millions of dollars at AI projects will fail because they have not taken the time to think through the problem and answer the right questions for successful implementation.
They believe that all data has value and the best way to mine that value is through an AI-created dashboard. Dashboards are great, but to bring the term full-circle back to the automotive world, no one ever bought a car because it has a great dashboard.
Dashboards only tell the surface story of your data: Throwing around general, surface-level data will not help solve the ultimate problem. The real question that should be asked is: “Which data can solve the current problem?” This is crucial in determining what a company’s highest priority projects should be, and then determining corresponding KPIs and revenue streams.
Increasing revenue and using mission critical data that ties back to success is of the upmost importance. With AI technology, first impressions go a long a way, and companies cannot afford their first or few AI projects to fail. This can set a company back with its customers and against its competition, thereby discouraging upper management to pursue other AI projects. You have one shot, and unless AI projects are aligned with company goals, revenue or KPIs, an AI implementation project won’t be effective. These projects must be business-focused and aligned with other priorities.
- Insufficient Data Expertise
It is absolutely imperative that data scientists working on an AI project have actual experience in the field. Business street smarts and experience must be tempered with an AI background. There are a glut of statisticians now involved with AI, but they don’t often know what they are talking about because they aren’t focused on the problem-solving aspect.
The right lead will have prior experience in creating AI projects, but will have also worked at other companies where they made critical mistakes and learned from them. Often, academic data scientists without experience will create surface level solutions that are a waste of time and resources for a company.
AI projects can be unnecessarily overcomplicated when the right steps are not taken into consideration in the very beginning.
- Communication Roadblocks
Data scientists enjoy focusing on complicated verbiage and digging deep into problem solving. While this works in certain situations, a very different conversation needs to happen when implementing AI. AI projects need to be aligned with the highest priorities and critical objectives within the company, and the best implementation plan is often to work backwards from the end goal and then determine how you can get there.
Equally critical to the success of any AI project is asking the right questions at the right time:
- What does AI success look like for the problem at hand?
- Who will be using this system?
- What are you trying to solve?
- What is the need for the AI implementation and how will it help drive success?
- Do you understand the business need for the AI solution you’re creating?
- What is the ideal service level agreement (SLA)?
It’s important to prepare to fail before you start. First, think through how the data needs to be pulled and what the right data points are before starting the project.
Next, get full commitment from all parties across the board with an outline of goals before a project starts. Finally, go through what possible roadblocks could stop success and what the overall cost will be to implement your AI data project.
Getting clarity on the business problem first enables employees to begin using the AI system effectively. In turn, this will help them understand the purpose of the system, what they need to aid their roles, and what will help them be more successful.
The reality is that data constantly changes, so being nimble in obtaining data quickly allows teams to get the information they want when they need it. AI projects often fail because the data has to be labeled, which can be a slow and laborious process. But when done in the most efficient way, AI can do the hard work.
About the Author
Dr. Akli Adjaoute, CEO of Brighterion, is a renowned academic, speaker, and writer on the subjects of AI, ML and security. He served Director of Cognitive Science and Artificial Intelligence at EPITA (Ecole Pour l’Informatique et les Techniques Avancees), one of the leading computer science universities in Europe. Prior to founding Brighterion, Dr. Adjaoute was the founder, President and CEO of Cognitix Technologies, previously known as Conception En Intelligence Artificielle, developer of MINDSuite.He has a PhD in artificial intelligence from University Pierre & Marie Curie. Dr. Adjaoute’s doctorate was awarded the “Mention Spéciale” by the Tribune des Mémoires et Thèses and he was the first to bring Smart Agent Technology to the market. Dr. Adjaoute served as an adjunct professor in Artificial Intelligence and Business Intelligence at the University of San Francisco (USF).
Recognized as a leading figure in the international cognitive science community, Dr. Akli Adjaoute is the recipient of numerous awards for his development of artificial intelligence (AI) and machine learning (ML) applications that provide real-time results for mission critical information.
Dr. Adjaoute is the Chief Executive Officer of Brighterion, Inc., which he founded in 2000. Dr. Adjaoute drove the creation of this award-winning AI platform that secures 100 billion transactions annually for many of the world’s leading organizations and governments. Focusing on payments, compliance, financial markets, security and defense, healthcare, Internet of Things, and marketing, Brighterion serves 74/100 of the largest U.S. banks and more than 2,000 customers worldwide.
Acquired by Mastercard in 2017, Brighterion continues to operate independently.