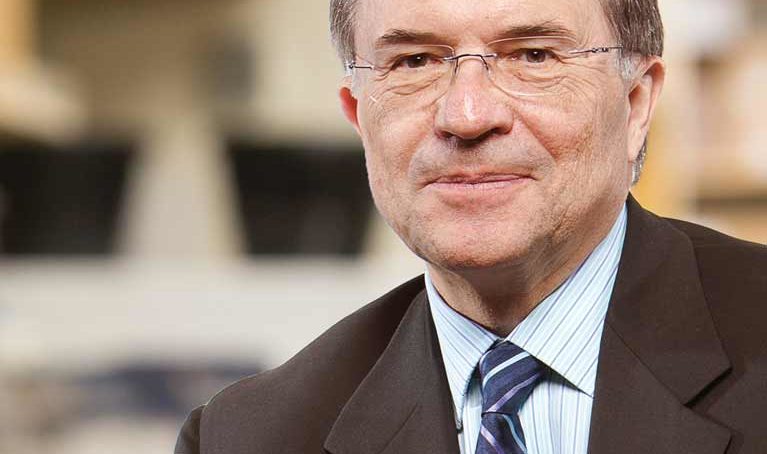
In his new book, The Deep Learning Revolution, Terry Sejnowski explains how deep learning went from being an arcane academic field to a disruptive technology in the information economy.
Sejnowski played an important role in the founding of deep learning, as one of a small group of researchers in the 1980s who challenged the prevailing logic-and-symbol based version of AI. The new version of AI Sejnowski and others developed, which became deep learning, is fueled instead by data. Deep networks learn from data in the same way that babies experience the world, starting with fresh eyes and gradually acquiring the skills needed to navigate novel environments. Learning algorithms extract information from raw data; information can be used to create knowledge; knowledge underlies understanding; understanding leads to wisdom. Someday a driverless car will know the road better than you do and drive with more skill; a deep learning network will diagnose your illness; a personal cognitive assistant will augment your puny human brain. It took nature many millions of years to evolve human intelligence; AI is on a trajectory measured in decades. Sejnowski prepares us for a deep learning future.
***
The age of cognitive computing is dawning. Soon we will have self-driving cars that drive better than we do. Our homes will recognize us, anticipate our habits and alert us to visitors. Kaggle, a crowdsourcing website recently bought by Google, ran a $1 million contest for a program to detect lung cancer in CT scans and is running a $1.5 million contest for the Department of Homeland Security for a program to detect concealed items in body scans at airports. With cognitive computing, doctor’s assistants will be able to diagnose even rare diseases and raise the level of medical care. There are thousands of applications like these, and many more have yet to be imagined. Some jobs will be lost; others will be created. Although cognitive computing technologies are disruptive and will take time for our society to absorb and adjust to, they aren’t existential threats. On the contrary, we are entering an era of discovery and enlightenment that will make us smarter, live longer, and prosper.
I was a speaker at an IBM-sponsored cognitive computing conference in San Francisco in 2015. IBM was making a big investment in Watson, a program based on collections of large databases of facts about everything from history to popular culture that could be interrogated with a wide range of algorithms using a natural language interface. Ken Jennings had won 74 games in a row over 192 days on Jeopardy!, the longest winning streak in the history of the game show. When Watson nonetheless beat Jennings on Jeopardy! in 2011, the world took notice.
In the taxi from my hotel to the conference, I overheard two IBM executives in the back of the car talking shop. IBM was rolling out a platform around Watson that could be used to organize and answer questions from unstructured databases in specialized areas such as health and financial services. Watson can answer questions and make recommendations that are based on more data than any human could possibly know, although, of course, as with other machine learning programs, it still takes humans to ask the questions and choose among the recommendations made.
IBM had long since parted with its hardware division, and its computer services division was no longer competitive. By banking on Watson, IBM was counting on its software division to help replace a $70 billion revenue stream. The company has invested $200 million in a new global headquarters for its Watson Internet of Things business in Munich, one of IBM’s largest investments ever in Europe in response to growing demand from more than 6,000 customers who want to transform their operations with artificial intelligence—and only part of the company’s global plan to invest $3 billion in cognitive computing. But many other companies are also making major investments into AI and it is too early to say which bets will be winners, and who will be the losers.
Life in the Twenty-First Century
In traditional medicine, the same treatment was typically made to fit all patients suffering from a given condition or illness, but now, thanks to cognitive computing, treatment has become personalized and precise. The progress of melanomas, which used to be death sentences, can now be halted and even reversed in many patients by sequencing a patient’s cancer cells and designing a specific cancer immunotherapy treatment for that person’s cancer. Although this treatment today costs $250,000, it will eventually be affordable for almost every melanoma patient since the base cost of sequencing a patient’s cancer genome is only a few thousand dollars and the cost of the necessary monoclonal antibodies only a few hundred dollars. Medical decision making will get better and less expensive once sufficient data have been amassed from patients with a wide range of mutations and outcomes. Some lung cancers are also treatable with the same approach. Pharmaceutical companies are investing in cancer immunotherapy research, and many other cancers may soon be treatable. None of this would have been possible without machine learning methods for analyzing huge amounts of genetic data.
I served on the committee that advised the director of the National Institutes of Health (NIH) on recommendations for the U.S. Brain Research through Advancing Innovative Neurotechnologies (BRAIN) Initiative. Our report emphasized the importance of probabilistic and computational techniques for helping us interpret data being generated by the new neural recording techniques. Machine learning algorithms are now used to analyze simultaneous recordings from thousands of neurons, to analyze complex behavioral data from freely moving animals and to automate reconstructions of 3D anatomical circuits from serial electron microscopic digital images. As we reverse engineer brains, we will uncover many new algorithms discovered by nature.
The NIH has funded basic research into neuroscience over the last fifty years, but the trend is to direct more and more of its grant support toward translational research with immediate health applications. Although we certainly want to translate what already has been discovered, if we do not also fund new discoveries now, there will be little or nothing to translate to the clinic fifty years from now. This also is why it is so important to start research programs like the BRAIN Initiative today in order to find future cures for debilitating brain disorders like schizophrenia and Alzheimer’s disease.
The Future of Identity
In 2006, the social security numbers and birth dates of 26.5 million veterans were stolen from the home of a Department of Veterans Affairs employee. Hackers wouldn’t even have had to decrypt the database since the Veterans Administration was using social security numbers as the identifiers for veterans in their system. With a social security number and a birth date, a hacker could have stolen any of their identities.
In India, more than a billion citizens can be uniquely identified by their fingerprints, iris scans, photographs, and twelve-digit identity numbers (three digits more than social security numbers). India’s Aadhaar is the world’s largest biometric identity program. In the past, an Indian citizen who wanted a public document faced endless delays and numerous middlemen, each requiring tribute. Today, with a quick bioscan, a citizen can obtain subsidized food entitlements and other welfare benefits directly, and many poor citizens who lack birth certificates now have a portable ID that can be used to identify them anytime and anywhere in seconds. Identity theft that siphoned off welfare support has been stopped. A person’s identity cannot be stolen, unless the thief is prepared to cut off the fingers and enucleates the eyes of that person.
The Indian national registry was a seven-year project for Nandan Nilekani, the billionaire and cofounder of Infosys, an outsourcing company. Nilekani’s massive digital database has helped India to leapfrog ahead of many developed countries. According to Nilekani: “A small, incremental change multiplied by a billion is a huge leap. … If a billion people can get their mobile phone in 15 minutes instead of one week that’s a massive productivity injection into the economy. If a million people get money into their bank accounts automatically that’s a massive productivity leap in the economy.”
The advantages of having a digital identity database are balanced by a loss of privacy, especially when the biometric ID is linked to other databases, such as bank accounts, medical records, and criminal records, and other public programs such as transportation. Privacy issues are already paramount in the United States and many other countries where databases are being linked, even when their data are anonymized. Thus our cell phones already track our whereabouts, whether we want them to or not.
The Rise of Social Robots
Movies often depict artificial intelligence as a robot that walks and talks like a human. Don’t expect an AI that looks like the German-accented Terminator in the 1984 science fiction/fantasy film The Terminator. But you will communicate with AI voices like Samantha’s in the 2013 romance/science fiction film Her and interact with droids like R2-D2 and BB-8 in the 2017 science fiction/fantasy film Star Wars: The Force Awakens. AI is already a part of everyday life. Cognitive appliances like Alexa in the Amazon Echo speaker already talk to you, happy to help make your life easier and more rewarding, just like the clocks and tea setting in the 2017 fantasy/romance film Beauty and the Beast. What will it be like to live in a world that has such creatures in it? Let’s take a look at our first steps toward social robots.
The current advances in artificial intelligence have primarily been on the sensory and cognitive sides of intelligence, with advances on motor and action intelligence yet to come. I sometimes begin a lecture by saying that the brain is the most complex device in the known universe, but my wife, Beatrice, who is a medical doctor, often reminds me that the brain is only a part of the body, which is even more complex than the brain, although the body’s complexity is different, arising from the evolution of mobility.
Our muscles, tendons, skin, and bones actively adapt to the vicissitudes of the world, to gravity, and to other human beings. Internally, our bodies are marvels of chemical processing, transforming foodstuffs into exquisitely crafted body parts. They are the ultimate 3D printers, which work from the inside out. Our brains receive inputs from visceral sensors in every part of our bodies, which constantly monitor the internal activity, including at the highest levels of cortical representation, and make decisions on internal priorities and maintain a balance between all the competing demands. In a real sense, our bodies are integral parts of brains, which is a central tenet of embodied cognition.
RUBI Project
Javier Movellan is from Spain and was a faculty member and co-director of the Machine Perception Laboratory at the Institute for Neural Computation at UC, San Diego. He believed that we would learn more about cognition by building robots that interact with humans than by conducting traditional laboratory experiments. He built a robot baby that smiled at you when you smile at it, which was remarkably popular with passersby. Among Javier’s conclusions after studying babies interacting with their mothers was that babies maximize smiles from their moms while minimizing their own effort.
Javier’s most famous social robot, Rubi, looks like a Teletubby, with an expressive face, eyebrows that rise to show interest, camera eyes that move around, and arms that grasp. In the Early Childhood Education Center at UCSD, 18-month-old toddlers interacted with Rubi using the tablet that serves as Rubi’s tummy.
Toddlers are difficult to please. They have very short attention spans. They play with a toy for a few minutes, then lose interest and toss it away. How would they interact with Rubi? On the first day, the boys yanked off Rubi’s arms, which, for the sake of safety, were not industrial strength. After some repair and a software patch, Javier tried again. This time, the robot was programmed to cry out when its arms were yanked. This stopped the boys, and made the girls rush to hug Rubi. This was an important lesson in social engineering.
Toddlers would play with Rubi by pointing to an object in the room, such as a clock. If Rubi did not respond by looking at that object in a narrow window of 0.5 to 1.5 seconds, the toddlers would lose interest and drift away. Too fast and Rubi was too mechanical; too slow and Rubi was boring. Once a reciprocal relationship was formed, the children treated Rubi like a sentient being rather than a toy. When the toddlers became upset after Rubi was taken away (to the repair shop for an upgrade), they were told instead that Rubi was feeling sick and would stay home for the day. In one study, Rubi was programmed to teach toddlers Finnish words, which they picked up with as much alacrity as they had English words; a popular song was a powerful reinforcer.
One of the concerns about introducing Rubi into a classroom setting was that teachers would feel threatened by a robot that might someday replace them. But quite the opposite occurred: teachers welcomed Rubi as an assistant that helped keep the class under control, especially when they had visitors in the classroom. An experiment that could have revolutionized early education was the “thousand Rubi project.” The idea was to mass produce Rubis, place them in a thousand classrooms, and collect data over the Internet from thousands of experiments each day. One of the problems with educational studies is that what works in one school may not work in another because there are so many differences between schools, especially between teachers. A thousand Rubis could have tested many ideas for how to improve educational practice and could have probed the differences between schools serving different socioeconomic groups around the country. Although resources never materialized to run the project, it’s still a great idea, which someone should pursue.
Two-legged robots are unstable and require a sophisticated control system to keep them from falling over. And, indeed, it takes about twelve months before a baby biped human starts walking without falling over. Rodney Brooks wanted to build six-legged robots that could walk like insects. He invented a new type of controller that could sequence the actions of the six legs to move his robo-cockroach forward and remain stable. His novel idea was to let the mechanical interactions of the legs with the environment take the place of abstract planning and computation. He argued that, for robots to accomplish everyday tasks, their higher cognitive abilities should be based on sensorimotor interaction with the environment, not on abstract reasoning. Elephants are highly social, have great memories, and are mechanical geniuses, but they don’t play chess. In 1990, Brooks went on to found iRobot, which has sold more than 10 million Roombas to clean even more floors.
Industrial robots have stiff joints and powerful servomotors, which makes them look and feel mechanical. In 2008, Brooks started Rethink Robotics (acquired by HAHN Group on October 25, 2018), a company that built a robot called “Baxter” with pliant joints, so its arms could be moved around. Instead of having to write a program to move Baxter’s arms, each arm could be moved through the desired motions, and it would program itself to repeat the sequence of motions.
Movellan went one step further than Brooks and developed a robot baby called “Diego San” (manufactured in Japan), whose motors were pneumatic (driven by air pressure) and all of whose forty-four joints were compliant compared to the stiff torque motors used in most industrial robots. The motivation for making them so is that when we pick something up, every muscle in our bodies is involved to some extent (when we move only one joint at a time, we look like robots). This makes us better able to adapt to changing conditions of load and interaction with the world. The brain can smoothly control all of the degrees of freedom in the body—all the joints and muscles—at the same time and the goal of the Diego San project was to figure out how. Diego San’s face had twenty-seven moving parts and could express a wide range of human emotions. The movements made by the robot baby were remarkably lifelike. Although Javier had several successful robot projects to his name, Diego San was not one of them, however. He simply didn’t know how to make the robot baby perform as fluidly as a human baby.
Facial Expressions Are a Window into Your Soul
Imagine what it would be like to watch your iPhone as stock prices plummet and have it ask you why you’re upset. Your facial expressions are a window into the emotional state of your brain and deep learning can now see into that window. Cognition and emotion have traditionally been considered separate functions of the brain. It was generally thought that cognition was a cortical function and emotions were subcortical. In fact, there are subcortical structures that regulate emotional states, structures like the amygdala, which is engaged when the emotional levels are high, especially fear, but these structures interact strongly with the cerebral cortex. Engagement of the amygdala in social interaction, for example, will lead to a stronger memory of the event. Cognition and emotions are intertwined.
In the 1990s, I collaborated with Paul Ekman, a psychologist at UC, San Francisco, who is the world’s leading expert on facial expressions and the real-world inspiration for Dr. Cal Lightman in the TV drama series Lie to Me, though Paul is a lot nicer than Cal. Ekman went to Papua New Guinea to find out if preindustrial cultures responded emotionally with the same facial expressions that we do. He found six universal expressions of emotion in all the human societies he studied: happiness, sadness, anger, surprise, fear, and disgust. Since then other universal facial expressions have been suggested, but there is no universal agreement, and some expressions, like fear, are interpreted differently in a few isolated societies.
In 1992, Ekman and I organized a Planning Workshop on Facial Expression Understanding sponsored by the National Science Foundation. At the time, it was quite difficult to get support for research on facial expressions. Our workshop brought researchers from neuroscience, electrical engineering, and computer vision together with psychologists, which opened a new chapter in analyzing faces. It was a revelation to me that, despite how important the analysis of facial expressions could be for so many areas of science, medicine, and the economy, it was being neglected by funding agencies.
Ekman developed the Facial Action Coding System (FACS) to monitor the status of each of the forty-four muscles in the face. FACS experts trained by Ekman take an hour to label a minute of videos, one frame at a time. Expressions are dynamic and can extend for many seconds, but Ekman discovered that there were some expressions that lasted for only a few frames.
These “microexpressions” were emotional leaks of suppressed brains states and were often telling, sometimes revealing unconscious emotional reactions. Microexpressions of disgust during a marriage counseling session, for example, were a reliable sign that the marriage would fail.
In the 1990s, we used video recordings from trained actors who could control every muscle on their face, as could Ekman, to train neural networks with backprop to automate the FACS. In 1999, a network trained with backprop by my graduate student Marian Stewart Bartlett had an accuracy of 96 percent in the lab, with perfect lighting, fully frontal faces, and manual temporal segmentation to video, a performance good enough to merit an appearance by Marni and me on Good Morning America with Diane Sawyer on April 5, 1999. Marni continued to develop the Computer Expression Recognition Toolbox (CERT) as a faculty member in the Institute for Neural Computation at UC, San Diego, and as computers became faster, CERT approached real-time analysis, so that it could label the changing facial expressions in a video stream of a person.
In 2012, Marni Bartlett and Javier Movellan started a company called “Emotient” to commercialize the automatic analysis of facial expressions. Paul Ekman and I served on its Scientific Advisory Board. Emotient developed deep learning networks that had an accuracy of 96 percent in real time and with natural behavior, under a broad range of lighting conditions, and with nonfrontal faces. In one of Emotient’s demos, its networks detected within minutes that Donald Trump was having the highest emotional impact on a focus group in the first Republican primary debate. It took the pollsters days to reach the same conclusion and pundits months to recognize that emotional engagement was key to reaching voters. The strongest facial expressions in the focus group were joy followed by fear. Emotient’s deep learning networks also predicted which TV series would become hits months before the Nielsen ratings were published. Emotient was bought by Apple in January 2016, and Marni and Javier now work for Apple.
In the not too distant future, your iPhone may not only be asking you why you’re upset; it may also be helping you to calm down.
About the Author
Terrence J. Sejnowski holds the Francis Crick Chair at the Salk Institute for Biological Studies and is a Distinguished Professor at the University of California, San Diego. He was a member of the advisory committee for the Obama administration’s BRAIN initiative and is President of the Neural Information Processing (NIPS) Foundation. He has published twelve books, including (with Patricia Churchland) The Computational Brain (25th Anniversary Edition, MIT Press). His most recent book is The Deep Learning Revolution (The MIT Press, 2018), from which this article is excerpted.